By Samantha Schoenlank and Antonia Egli (Dublin City University)
This month, we continue to explore the transformative impact of technology on the renovation sector by covering RINNO’s newly published open-access book ‘Disrupting Buildings: Digitalisation and the Transformation of Deep Renovation’. Read on for insights from Chapter 6 ‘Digital Twins and Their Roles in Building Deep Renovation Life Cycle’ and dive into some key points to help understand digital twins in construction and renovation. This chapter exposes the various definitions of digital twins, illustrates the basic steps and relevant methods for creating a digital twin, explores of state-of-the-art deep learning methods for digital twins, and discusses some real-life use cases.
The construction sector, which often grapples with challenges like low productivity and environmental impact, stands at the brink of transformation through digitalisation. In this chapter, we delve into the potential of digital twins in addressing these challenges within the architecture, engineering, construction, and operations (AECO) sector. Digital twins, an emerging paradigm, offer a glimpse into smart buildings and cities, and bring about increased productivity and efficiency.
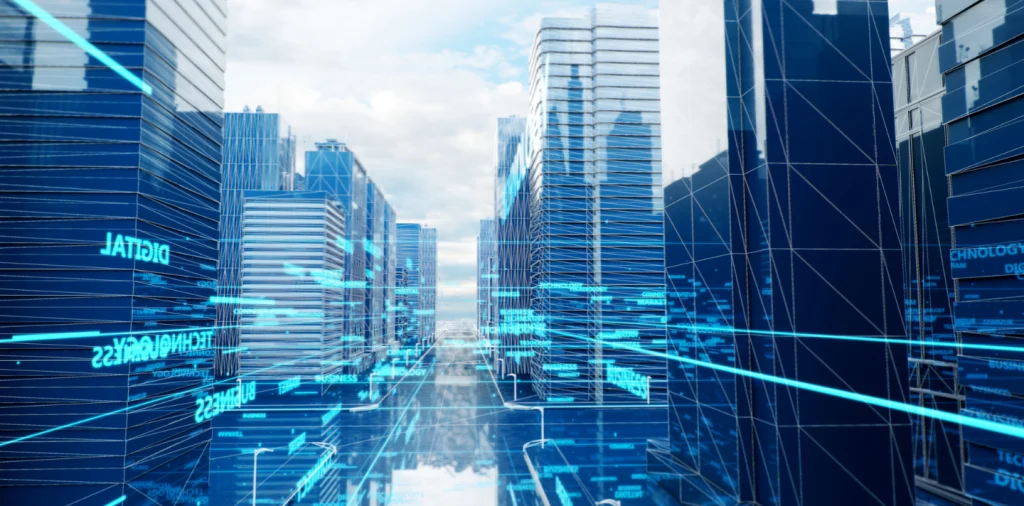
Twinning in AECO (credits: NEWFORMA)
Understanding Digital Twins
Within the realm of innovative technological paradigms, digital twins stand out as dynamic entities, encapsulating physical assets within the architecture, engineering, construction, and operations (AECO) sector. The concept of digital twins revolves around three fundamental elements: the tangible physical product, its virtual counterpart, and their integral connection enabling seamless data exchange.
Digital twins emerge as information repositories throughout an asset’s life cycle. The life cycle of a digital twin unfolds in three distinct stages: design, construction, and operation. Each stage contributes to the accumulation of valuable data, transforming the digital twin into an information repository with far-reaching implications.
- Design Stage: In the design stage, the asset’s conceptual plan takes shape. The digital twin, at this embryonic phase (“foetal digital twin”), encapsulates a wealth of product and process information. The creation of a geometric digital twin involves using cutting-edge technologies like laser scanning and photogrammetry. Multiple building information models (BIMs) are proposed, converging into a client-approved design file labelled “Design Intent.” This serves as a benchmark, guiding subsequent construction and maintenance endeavours.
- Construction Stage: As the asset transitions to the construction stage, the “child digital twin” emerges, incorporating off-site prefabricated assemblies and on-site constructed components. Simultaneously, the child digital twin captures as-built product information and as-performed process information, mirroring the asset’s evolving physical status. Changes are continually updated, facilitating progress monitoring and quality control.
- Operation Stage: Finally, in the operation stage, the “adult digital twin” attains an unchangeable status post-construction. The adult digital twin, now a mature repository, supports in-depth analyses of performance metrics such as energy consumption and component maintenance. Data collected during operations enriches the adult digital twin, fostering a holistic understanding of the asset’s long-term behaviour.
This dynamic evolution through a building’s life cycle positions digital twins as invaluable tools, offering a comprehensive narrative of the asset’s journey and enabling nuanced performance analysis at every stage.
Digital twins find diverse applications in the construction and renovation sectors, serving roles in progress monitoring, facilities management, asset condition monitoring, and sustainable development. From real-time progress monitoring during construction/renovation to predictive maintenance and IoT-driven facility management, digital twins showcase their versatility.
Challenges to Digital Twin Adoption
While the potential benefits of digital twins are undeniable, the road to adoption is not without hurdles. Although significant strides have been made, challenges persist particularly in the manual detection of geometric objects. Deep learning offers some support, such as a dearth of labelled datasets in the AECO domain, to bring about future seamless automation. Effort-intensive processes, particularly in reconstructing MEP (mechanical, electrical, plumbing) elements, equally pose challenges to the feasibility of digital twins. Automation efforts have primarily targeted large structural elements, leaving intricacies such as floor plans and object IDs to manual intervention. Overcoming these challenges is imperative to unlocking the full potential of digital twins.
Future Direction
‘Disrupting Buildings’ Chapter 6 offers a comprehensive exploration of digital twins in the built environment with a specific focus on building renovation. Acknowledging the potential benefits of digital twins, it also underscores the challenges in generating and updating digital twins efficiently. The need for ongoing research to support practical applications throughout a facility’s life cycle is emphasised, reflecting the evolving quest for precision and efficiency in digital twin implementation.
To learn more about deep renovation technologies in general, you can download the open access book ‘Disrupting Buildings: Digitalisation and the Transformation of Deep Renovation’ for free. To download the full open access book, ‘Disrupting Buildings,’ click here.
References
Adán, A., Quintana, B., Prieto, S. A., & Bosché, F. (2018). Scan-to-BIM for ‘secondary’ building components. Advanced Engineering Informatics, 37, 119–138. https://doi.org/10.1016/J.AEI.2018.05.001
Agapaki, E., & Brilakis, I. (2020). CLOI-NET: Class segmentation of industrial facilities’ point cloud datasets. Advanced Engineering Informatics, 45(November 2019). https://doi.org/10.1016/j.aei.2020.101121
Agapaki, E., & Brilakis, I. (2021). Instance segmentation of industrial point cloud data. Journal of Computing in Civil Engineering, 35(6). https://doi.org/10.1061/(asce)cp.1943-5487.0000972
Agapaki, E., Miatt, G., & Brilakis, I. (2018). Prioritizing object types for modelling existing industrial facilities. Automation in Construction, 96(September), 211–223. https://doi.org/10.1016/j.autcon.2018.09.011
Baek, F., Ha, I., & Kim, H. (2019). Augmented reality system for facility management using image-based indoor localization. Automation in Construction, 99(November 2018), 18–26. https://doi.org/10.1016/j.autcon.2018.11.034
Cao, Y., Song, X., & Wang, T. (2015). Development of an energy-aware intelligent facility management system for campus facilities. Procedia Engineering, 118, 449–456. https://doi.org/10.1016/j.proeng.2015.08.446
Chen, H., Hou, L., Zhang, G., & Moon, S. (2021). Development of BIM, IoT and AR/VR technologies for fire safety and upskilling. Automation in Construction, 125(September 2020), 103631. https://doi.org/10.1016/j.autcon.2021.103631
Chen, K., Yang, J., Cheng, J. C. P., Chen, W., & Li, C. T. (2020). Transfer learning enhanced AR spatial registration for facility maintenance management. Automation in Construction, 113(July 2019), 103135. https://doi.org/10.1016/j.autcon.2020.103135
Cheng, J. C. P., Chen, W., Chen, K., & Wang, Q. (2020). Data-driven predictive maintenance planning framework for MEP components based on BIM and IoT using machine learning algorithms. Automation in Construction, 112(December 2019), 103087. https://doi.org/10.1016/j.autcon.2020.103087
D’Urso, C. (2011). Information integration for facility management. IT Professional, 13(6), 48–53. https://doi.org/10.1109/MITP.2011.100
El Saddik, A. (2018). Digital twins: The convergence of multimedia technologies. IEEE Multimedia, 25(2), 87–92. https://doi.org/10.1109/MMUL.2018.023121167
Hu, Z., Brilakis, I., Karlinsky,L., Michaeli, T., & Nishino, K. (2023). Computer Vision – ECCV 2022 Workshops Tel Aviv Israel October 23–27 2022 Proceedings Part VII PriSeg: IFC-Supported Primitive Instance Geometry Segmentation with Unsupervised Clustering Springer Nature Switzerland Cham 196–211. https://doi.org/10.1007/978-3-031-25082-8_13.
Hu, Z., Fathy, Y., & Brilakis, I. (2022). Geometry updating for digital twins of buildings: A review to derive a new geometry-based object class hierarchy. Proceedings of the 2022 European Conference on Computing in Construction. https://doi.org/10.35490/ec3.2022.155
Hu, Z. Z., Zhang, J. P., Yu, F. Q., Tian, P. L., & Xiang, X. S. (2016). Construction and facility management of large MEP projects using a multi-scale building information model. Advances in Engineering Software, 100, 215–230. https://doi.org/10.1016/j.advengsoft.2016.07.006
Lu, R., Brilakis, I., & Middleton, C. R. (2019). Detection of structural components in point clouds of existing RC bridges. Computer-Aided Civil and Infrastructure Engineering, 34(3), 191–212. https://doi.org/10.1111/mice.12407
Maturana, D., & Scherer, S. (2015). VoxNet: A 3D Convolutional Neural Network for real-time object recognition. In IEEE International Conference on Intelligent Robots and Systems, 2015-Decem (pp. 922–928). IEEE. https://doi.org/10.1109/IROS.2015.7353481
Meerman, A., Lellek, V., & Serbin, D. (2014). The path to excellence: Integrating customer satisfaction in productivity measurement in Facility Management. International Journal of Facilities Management, 201–211.
Monszpart, A., Mellado, N., Brostow, G. J., & Mitra, N. J. (2015). RAPter: Rebuilding man-made scenes with regular arrangements of planes. ACM Transactions on Graphics, 34(4). https://doi.org/10.1145/2766995
Natephra, W., Motamedi, A., Fukuda, T., & Yabuki, N. (2017). Integrating building information modeling and virtual reality development engines for building indoor lighting design. Visualization in Engineering, 5(1). https://doi.org/10.1186/s40327-017-0058-x
Ochmann, S., Vock, R., Wessel, R., & Klein, R. (2016). Automatic reconstruction of parametric building models from indoor point clouds. Computers and Graphics (Pergamon), 54, 94–103. https://doi.org/10.1016/j.cag.2015.07.008
Oesau, S., Lafarge, F., & Alliez, P. (2014). Indoor scene reconstruction using feature sensitive primitive extraction and graph-cut. ISPRS Journal of Photogrammetry and Remote Sensing, 90, 68–82. https://doi.org/10.1016/j.isprsjprs.2014.02.004
Pan, Y., Braun, A., Borrmann, A., & Brilakis, I. (2021). Void-growing: A novel Scan-to-BIM method for Manhattan world buildings from point cloud. Proceedings of the 2021 European Conference on Computing in Construction, 2(2018), 312–321. https://doi.org/10.35490/ec3.2021.162
Pan, Y., Braun, A., Brilakis, I., & Borrmann, A. (2022). Enriching geometric digital twins of buildings with small objects by fusing laser scanning and AI-based image recognition. Automation in Construction, 140(February), 104375. https://doi.org/10.1016/j.autcon.2022.104375
Perez-Perez, Y., Golparvar-Fard, M., & El-Rayes, K. (2021). Scan2BIM-NET: Deep learning method for segmentation of point clouds for Scan-to-BIM. Journal of Construction Engineering and Management, 147(9). https://doi.org/10.1061/(asce)co.1943-7862.0002132
Qi, C., Yi, L., Su, H., & Guibas, L. (2017b). PointNet++: Deep hierarchical feature learning on. In NIPS’17: Proceedings of the 31st International Conference on Neural Information Processing Systems, Dec (pp. 5105–5114). ACM Digital Library.
Qi, C. R., Su, H., Mo, K., & Guibas, L. J. (2017a). PointNet: Deep learning on point sets for 3D classification and segmentation. In Proceedings—30th IEEE Conference on Computer Vision and Pattern Recognition, CVPR 2017, 2017-Janua (pp. 77–85). IEEE. https://doi.org/10.1109/CVPR.2017.16
Sacks, R., Brilakis, I., Pikas, E., Xie, H. S., & Girolami, M. (2020). Construction with digital twin information systems. Data-Centric Engineering, 1. https://doi.org/10.1017/dce.2020.16
Sanchez, V., & Zakhor, A. (2012). Planar 3D modeling of building interiors from point cloud data. In Proceedings—International Conference on Image Processing, ICIP (pp. 1777–1780). IEEE. https://doi.org/10.1109/ICIP.2012.6467225
Stambler, A., & Huber, D. (2015). Building modeling through enclosure reasoning. In Proceedings—2014 International Conference on 3D Vision Workshops, 3DV 2014 (pp. 118–125). ACM Digital Library. https://doi.org/10.1109/3DV.2014.65
Tao, F., Zhang, H., Liu, A., & Nee, A. Y. C. (2019). Digital twin in industry: State-of-the-Art. IEEE Transactions on Industrial Informatics, 15(4), 2405–2415. https://doi.org/10.1109/TII.2018.2873186
Tran, H., Khoshelham, K., Kealy, A., & Díaz-Vilariño, L. (2019). Shape grammar approach to 3D modeling of indoor environments using point clouds. Journal of Computing in Civil Engineering, 33(1). https://doi.org/10.1061/(asce)cp.1943-5487.0000800
Xiao, J., & Furukawa, Y. (2014). Reconstructing the world’s museums. International Journal of Computer Vision, 110(3), 243–258. https://doi.org/10.1007/s11263-014-0711-y
Zhang, Y., Liu, H., Kang, S. C., & Al-Hussein, M. (2020). Virtual reality applications for the built environment: Research trends and opportunities. Automation in Construction, 118(May), 103311. https://doi.org/10.1016/j.autcon.2020.103311