By Samantha Schoenlank and Antonia Egli (Dublin City University)
In our quest to explore the transformative impact of technology on the renovation and construction sectors, we continue with the RINNO blog series focusing on the open-access book ‘Disrupting Buildings: Digitalisation and the Transformation of Deep Renovation.’ This month, we bring you insights from Chapter 5 ‘Big Data and Analytics in the Deep Renovation Life Cycle.’ This chapter delves into the intersection of big data and deep renovation, shedding light on how big data and analytics can revolutionise decision-making in building stock retrofits.
The renovation and construction industries are experiencing a paradigm shift brought about by the proliferation of heterogeneous data. With the accessibility of technologies like sensors and the Internet of Things (IoT), data-driven decision-making is becoming integral, especially in the context of deep renovation. Chapter 5 defines big data and analytics in the deep renovation life cycle and explores the impact of machine learning (ML) and artificial intelligence (AI) on decision-making processes.
Categorising the Power of Big Data in Deep Renovation
The transformative power of big data in deep renovation lies in its ability to leverage vast amounts of data for more informed decision-making. Big data analytics takes centre stage and can be categorised into four distinct types: descriptive, diagnostic, predictive, and prescriptive analytics. Each plays a crucial role in interpreting and utilising data to enhance operational performance and decision-making in renovation and construction.
Descriptive analytics lays the groundwork by unveiling current and past trends, while diagnostic analytics digs deep to explain the causes behind specific outcomes. Predictive analytics, with the use of ML and statistical modelling, estimates outcomes, and prescriptive analytics optimises operational processes using insights gleaned from previous analyses.
Big Data Use Cases and Applications Reshaping Deep Renovation
The construction landscape is witnessing a transformative wave driven by big data engineering, prominently featuring Building Information Modelling (BIM). Horizontal Scaling Platforms (HSPs) and Vertical Scaling Platforms (VSPs) play pivotal roles, contributing to waste management, profitability performance measurement, smart road construction, and more. In parallel, deep learning-based applications, including flood detection and project delay risk prediction, showcase the integration of AI and ML in construction and renovation.
Within the realm of deep renovation, AI and ML find synergy with BIM and Industry Foundation Classes (IFC), propelling advancements in sustainable architecture, energy-efficient building design, and environmental impact mitigation. Deep renovation can thus emerge as a key driver for reducing greenhouse gas (GHG) emissions, with BIM and IFC enhancing both decision-making processes and the energy efficiency of retrofitted buildings.
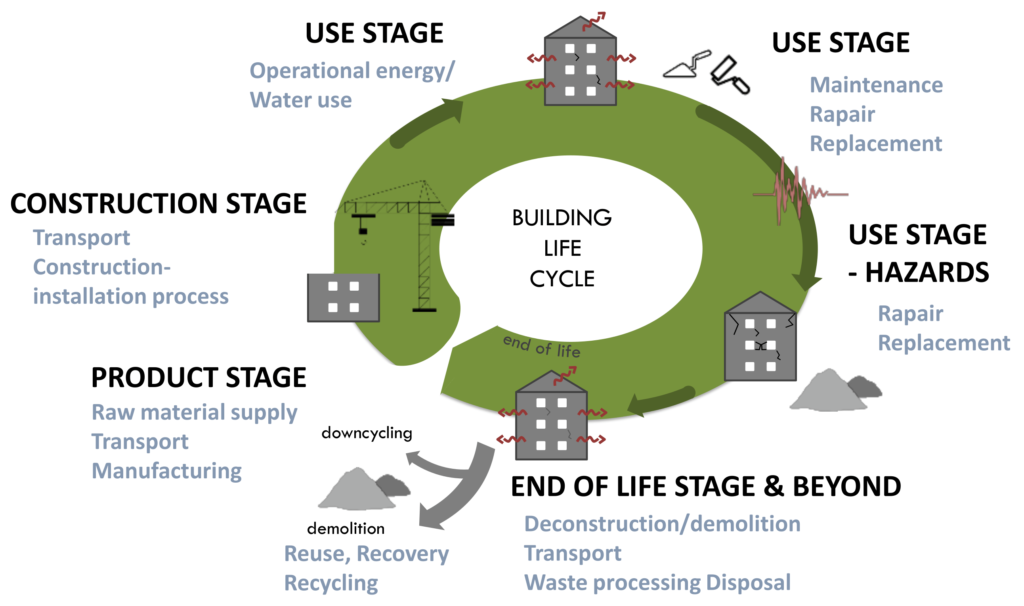
Building life cycle (credits: Zanni et al.)
Advantages & Benefits vs. Challenges & Barriers of Big Data Analytics
The literature reveals a myriad of opportunities for big data adaptation in deep renovation, spanning generative design, clash detection, performance prediction models, visual analytics, social networking, and personalised services. Beyond these applications, big data analytics in construction and renovation leads to tangible benefits, including improved efficiency and a reduction in environmental impact.
However, challenges and barriers also exist, ranging from human factors to technological integration issues, organisational barriers, and specific data-related challenges like data security and privacy. The potential for future developments is nonetheless immense. The integration of big data promises to benefit construction companies and stakeholders, fostering long-term infrastructure and preventing errors for better construction and renovation outcomes.
Future Developments in Big Data
The transformative power of big data in deep renovation lies in its ability to leverage vast amounts of data for more informed decision-making. While the construction and renovation sectors have made significant strides in adopting big data technologies, the journey towards widespread commercialisation is ongoing. Future developments may focus on the global commercialisation of big data analytics for deep renovation, construction waste simulation tools, linked building data platforms, and big data-driven BIM systems. As the industry continues to evolve, big data is poised to play a central role in shaping the future of construction research.
As we embrace this data-driven revolution, the construction industry stands at the cusp of unprecedented possibilities, where every byte of information contributes to a more efficient, sustainable, and innovative future. If you wish to explore further, we encourage you to delve into Chapter 5 of the book for a comprehensive understanding and additional multidimensional applications.
To learn more about deep renovation technologies in general, you can download the open access book ‘Disrupting Buildings: Digitalisation and the Transformation of Deep Renovation’ for free. In the book, we explore various digital innovations disrupting and transforming the construction sector. To download the full open access book, ‘Disrupting Buildings,’ click here.
References
Androutsopoulos, A., Geissler, S., Charalambides, A. G., Escudero, C. J., Kyriacou, O., & Petran, H. (2020). Mapping the deep renovation possibilities of European buildings. IOP Conference Series: Earth and Environmental Science, 410(1), 12056. https://doi.org/10.1088/1755-1315/410/1/012056
Avramidou, A., & Tjortjis, C. (2021). In I. Maglogiannis, J. Macintyre, & L. Iliadis (Eds.), Predicting CO2 emissions for buildings using regression and classification BT—Artificial Intelligence applications and innovations (pp. 543–554). Springer International Publishing.
Bilal, M., Oyedele, L. O., Akinade, O. O., Ajayi, S. O., Alaka, H. A., Owolabi, H. A., Qadir, J., Pasha, M., & Bello, S. A. (2016b). Big Data architecture for Construction Waste Analytics (CWA): A conceptual framework. Journal of Building Engineering, 6, 144–156.
Bilal, M., Oyedele, L. O., Kusimo, H. O., Owolabi, H. A., Akanbi, L. A., Ajayi, A. O., Akinade, O. O., & Delgado, J. M. D. (2019). Investigating profitability performance of construction projects using Big Data: A project analytics approach. Journal of Building Engineering, 26, 100850.
Bilal, M., Oyedele, L. O., Qadir, J., Munir, K., Ajayi, S. O., Akinade, O. O., Owolabi, H. A., Alaka, H. A., & Pasha, M. (2016a). Big Data in the construction industry: A review of present status, opportunities, and future trends. Advanced Engineering Informatics, 30(3), 500–521. https://doi.org/10.1016/j.aei.2016.07.001
Cluett, R., & Amann, J. (2014). Residential deep energy retrofits. March, 64. www.aceee.org
Curtis, C. (2020). Architecture at scale: Reimagining one-off projects as building platforms. Architectural Design, 90(2), 96–103.
D’Agostino, D., Zangheri, P., & Castellazzi, L. (2017). Towards nearly zero energy buildings in Europe: A focus on retrofit in non-residential buildings. Energies, 10(1), 117. https://doi.org/10.3390/en10010117
D’Oca, S., Ferrante, A., Ferrer, C., Pernetti, R., Gralka, A., Sebastian, R., & Op ‘t Veld, P. (2018). Technical, financial, and social barriers and challenges in deep building renovation: Integration of lessons learned from the H2020 cluster projects. Buildings, 8(12). https://doi.org/10.3390/buildings8120174
Filippidou, F., Nieboer, N., & Visscher, H. (2017). Are we moving fast enough? The energy renovation rate of the Dutch non-profit housing using the national energy labelling database. Energy Policy, 109, 488–498. https://doi.org/10.1016/j.enpol.2017.07.025
Gbadamosi, A.-Q., Oyedele, L., Mahamadu, A.-M., Kusimo, H., Bilal, M., Delgado, J. M. D., & Muhammed-Yakubu, N. (2020). Big Data for Design Options Repository: Towards a DFMA approach for offsite construction. Automation in Construction, 120, 103388.
Glumac, B., Reuvekamp, S., Han, Q., & Schaefer, W. (2013). Tenant participation in sustainable renovation projects: Using AHP and case studies. Journal of Energy Technologies and Policy—Special Issue for International Conference on Energy, Environment and Sustainable Economy (EESE 2013), 3(11), 16–26.
Gondia, A., Siam, A., El-Dakhakhni, W., & Nassar, A. H. (2020). Machine learning algorithms for construction projects delay risk prediction. Journal of Construction Engineering and Management, 146(1), 4019085.
Han, Z., & Wang, Y. (2017). The applied exploration of Big Data technology in prefabricated construction project management. In ICCREM 2017 (pp. 71–78). ASCE Library.
Huang, X. (2021). Application of BIM Big Data in construction engineering cost. Journal of Physics: Conference Series, 1865(3), 32016.
Ismail, S. A., Bandi, S., & Maaz, Z. N. (2018). An appraisal into the potential application of Big Data in the construction industry. International Journal of Built Environment and Sustainability, 5(2).
Koukaras, P., Bezas, N., Gkaidatzis, P., Ioannidis, D., Tzovaras, D., & Tjortjis, C. (2021a). Introducing a novel approach in one-step ahead energy load forecasting. Sustainable Computing: Informatics and Systems, 32, 100616. https://doi.org/10.1016/j.suscom.2021.100616
Koukaras, P., Gkaidatzis, P., Bezas, N., Bragatto, T., Carere, F., Santori, F., Antal, M., Tjortjis, C., & Tzovaras, D. (2021b). A tri-layer optimization framework for day-ahead energy scheduling based on cost and discomfort minimization. Energies, 14(12), 3599. https://doi.org/10.3390/en14123599
Koukaras, P., & Tjortjis, C. (2019). Social media analytics, types and methodology. In Machine learning paradigms (pp. 401–427). Springer. https://doi.org/10.1007/978-3-030-15628-2_12
Kousis, A., & Tjortjis, C. (2021). Data mining algorithms for smart cities: A bibliometric analysis. Algorithms, 14(8), 242. https://doi.org/10.3390/a14080242
Loyola, M. (2018). Big Data in building design: A review. Journal of Information Technology in Construction, 23, 259–284.
Lu, W., Chen, X., Ho, D. C. W., & Wang, H. (2016). Analysis of the construction waste management performance in Hong Kong: The public and private sectors compared using Big Data. Journal of Cleaner Production, 112, 521–531.
Lynn, T., Rosati, P., Egli, A., Krinidis, S., Angelakoglou, K., Sougkakis, V., Tzovaras, D., Kassem, M., Greenwood, D., & Doukari, O. (2021). Rinno: Towards an open renovation platform for integrated design and delivery of deep renovation projects. Sustainability (Switzerland), 13(11). https://doi.org/10.3390/su13116018
Maqsoom, A., Aslam, B., Gul, M. E., Ullah, F., Kouzani, A. Z., Mahmud, M. A. P., & Nawaz, A. (2021). Using multivariate regression and ANN models to predict properties of concrete cured under hot weather. Sustainability, 13(18), 10164.
Marinakis, V. (2020). Big Data for energy management and energy-efficient buildings. Energies, 13(7). https://doi.org/10.3390/en13071555
Mehmood, M. U., Chun, D., Zeeshan, H., Jeon, G., & Chen, K. (2019). A review of the applications of artificial intelligence and Big Data to buildings for energy-efficiency and a comfortable indoor living environment. Energy and Buildings, 202, 109383. https://doi.org/10.1016/j.enbuild.2019.109383
Mulero-Palencia, S., Álvarez-Díaz, S., & Andrés-Chicote, M. (2021). Machine learning for the improvement of deep renovation building projects using as-built bim models. Sustainability (Switzerland), 13(12). https://doi.org/10.3390/su13126576
Munawar, H. S., Ullah, F., Qayyum, S., & Heravi, A. (2021). Application of deep learning on UAV-based aerial images for flood detection. Smart Cities, 4(3), 1220–1242.
Rahimian, F. P., Seyedzadeh, S., Oliver, S., Rodriguez, S., & Dawood, N. (2020). On-demand monitoring of construction projects through a game-like hybrid application of BIM and machine learning. Automation in Construction, 110, 103012.
Rajaraman, V. (2016). Big DATA analytics. Resonance, 21(8), 695–716. https://doi.org/10.1007/s12045-016-0376-7
Sharif, M., Mercelis, S., Van Den Bergh, W., & Hellinckx, P. (2017). Towards real-time smart road construction: Efficient process management through the implementation of internet of things. Proceedings of the International Conference on Big Data and Internet of Thing, 2017, 174–180.
Shtern, M., Mian, R., Litoiu, M., Zareian, S., Abdelgawad, H., & Tizghadam, A. (2014). Towards a multi-cluster analytical engine for transportation data. 2014 International Conference on Cloud and Autonomic Computing, 2014, 249–257.
Tixier, A. J.-P., Hallowell, M. R., Rajagopalan, B., & Bowman, D. (2016). Application of machine learning to construction injury prediction. Automation in Construction, 69, 102–114.
Wang, D., Fan, J., Fu, H., & Zhang, B. (2018). Research on optimization of Big Data construction engineering quality management based on RNN-LSTM. Complexity, 2018.
Yousif, O. S., Zakaria, R. B., Aminudin, E., Yahya, K., Mohd Sam, A. R., Singaram, L., Munikanan, V., Yahya, M. A., Wahi, N., & Shamsuddin, S. M. (2021). Review of Big Data integration in construction industry digitalization. Frontiers in Built Environment, 7(November), 1–13. https://doi.org/10.3389/fbuil.2021.770496