By Antonia Egli (Dublin City University)
Building simulation is a technique that uses computational, mathematical, and machine learning models to represent the physical characteristics, expected or actual operation, and control strategies of a building and its energy systems. The RINNO blog series on our recently published open access book ‘Disrupting Buildings: Digitalisation and the Transformation of Deep Renovation’ moves to Chapter 4: Building Performance Simulation this week with Asimina Dimara, Prof. Stelios Krinidis, Dr. Dimosthenis Ioannidis, and Dr. Dimitrios Tzovaras. Read on for a better understanding of the building performance simulation domain focusing on significant use cases, widely used simulation tools, and challenges for implementation. For timely updates on this blog series, sign up to the RINNO newsletter or follow us on social media here.
Building simulation (BS) is the process of creating a digital replica of a building, while building performance simulation (BPS) is a model that evaluates how the building performs under real-life conditions (Mahdavi, 2020). During the replication process, digital copies of a whole building are created — its exterior and interior, and, in some cases, the building’s distinct parts (e.g., apartments and rooms). Simulations can be used in a number of tasks along the deep renovation life cycle, including: (a) integrating simulations with other knowledge-based systems to support decision-making, (b) using simulations to evaluate and compare design scenarios, (c) integrating simulations with real-time monitoring and diagnostic systems for building energy management and control, (d) integrating multiple simulation applications, and (e) using virtual reality (VR) to enable digital building design and operation experiences.
Understanding the Building Performance Simulation Process
BPS is a dynamic technique that is used to predict the behaviour of a building while optimising energy efficiency (Attia et al., 2013). The BPS process consists of five main phases. The main objective of this process is to define the best performance criteria and the most suitable actions by applying performance simulations. As a result, BPS can support in reducing the building’s environmental footprint while improving indoor environmental quality (IEQ). At the same time, if applied correctly, BPS may facilitate technological innovation and progress in building construction (Loonen et al., 2017). Once results are generated, they are evaluated against initial expectations and requirements.
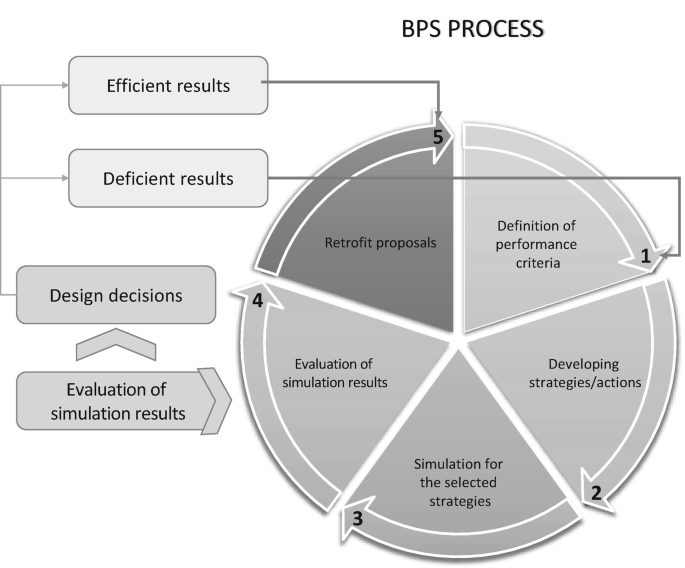
Building performance simulation process (Bramstoft et al., 2018)
To enable this process, mathematical and intelligent models and applications are exploited to recreate (i.e., simulate) various external and internal conditions while representing them in a virtual environment (Mahdavi, 2020). BPS makes it easier for different stakeholders (building managers, architects, engineers, etc.) to inspect and check salient points of the building’s life cycle (i.e., early design, construction, retrofitting, monitoring, inspection, and demolition) (Bramstoft et al., 2018).
Exploiting BS tools and applications is faster, safer, and less expensive than producing a real use case scenario. It supports product and system testing without having to build them in real life and is often less time-consuming and costly while also being safer. Moreover, BPS may be exploited for identifying building problems by replicating and producing different conditions. It can also be used to evaluate and compare design scenarios – for example, BPS provides the ability to examine various solutions during the design stage, like the efficiency of the building’s equipment and integration, therefore reducing development time and energy consumption and emissions. Moreover, BPS may facilitate the optimisation of thermal and visual comfort by simulating the building’s fenestration and massing. This process may also be deployed in the early stages while designing the façade. BPS also enables the simulation of heating, ventilation, and air conditioning (HVAC) systems to define the optimal setup. Finally, it may be used to model specific changes to check how the building reacts in the short or long term (Fernandez-Antolin et al., 2022).
BPS energy models are applied in a number of real-life applications. These include, for example, energy conservation, energy monitoring, energy savings, and fault detection. Simulations may include load and energy simulation, energy management simulation, virtual reality simulations, and a wide range of other simulations based on stakeholders’ needs (Martin, 2022).
Integrating Building (Performance) Simulations
Integrating Simulations with Other Knowledge-Based Decision Support Systems
Knowledge-based systems employ numerous techniques such as statistical analysis, artificial intelligence methods, knowledge and data visualisation, and engineering (Alor-Hernández & Valencia-García, 2017). These techniques have been developed to be integrated into heterogeneous systems including decision support systems (DSS), software agents (SAs), and knowledge engineering (KE). All these systems use prior knowledge to exclude, determine, and propose further knowledge. To deploy a knowledge-based system, an analysis of the building’s energy conservation is needed to inspect the building’s energy efficiency. In general, knowledge-based systems are the main component of building simulation and are mainly used as a process that emulates the simulation outcome.
Integrating Simulations with Real-Time Monitoring and Diagnostic Systems for Building Energy Management and Control
Radiation, conduction, and convection are mass and heat transfer phenomena that take place in a building and are the key inputs for energy and load simulations (Yu, 2019). As a result, every BS needs to take into account different mechanisms behind such phenomena. Heat and mass transfer are carried in the building by air movements and are embodied by the indoor air pressure stack between outdoor and indoor places (Vera, 2018). These phenomena are influenced by peoples’ activities, heating and cooling systems, and ventilation as well as building insulation and orientation (Puttur et al., 2022).
To better understand these processes, thermal and airflow models are applied to represent heat and mass transfers of buildings as they are significantly associated with the energy transfer (Tian, 2018b) and are used to calculate loads and simulate energy consumption (Tan et al., 2022). Frequently used models for simulating energy consumption include Computational Fluid Dynamics (CFD) models, zonal models, and multi-zone models (Laghmich et al., 2022). CFD models separate the building into cells to simulate load and energy consumption (Shen et al., 2020). A multi-zone model uses rooms as computational elements for the simulation, while a zonal model uses several zones by separating a room into smaller units (Yu, 2019).
Other models estimate a building’s energy consumption by exploiting physical models (Kampelis et al., 2020). While such mathematical or computational models are sufficiently precise, they require holistic building information (Oucquier et al., 2013). Furthermore, a unique model is required for each building. Another widely referenced approach to simulating building energy load is data-driven modelling (Bermeo-Ayerbe et al., 2022). These models use indoor monitoring and measurements (e.g., relative humidity, temperature, historical consumption data, historical load, and generations data) to predict energy consumption (Kampelis et al., 2020). A benchmarking analysis of various regression models using energy consumption suggests that, in many cases, these models are sufficiently accurate for building simulation and may be used as a generic solution (Dimara et al., 2021).
To manage the overall energy consumption of a building, various load controls are applied to manage both energy savings and comfort regulation. The energy load of all appliances in a building are simulated in order to build predictive models for energy consumption and deploy an accurate energy management strategy (Fanti et al., 2018). The main problem when trying to find optimal control states is to detect the best strategies for heating, cooling, ventilation, and lighting that result in energy savings while maintaining desirable indoor conditions for the occupants. As such, all possible energy load actions must be simulated accurately.
Integrating Multiple Simulation Applications
Most simulations require the deployment of multiple models, applications, and techniques to provide an overall building assessment. In general, deploying and integrating automated multi-simulation applications may produce significant advantages when compared to a single simulation. During this process, multiple models are integrated and their output is combined. For example, a comprehensive evaluation of the HVAC systems in a building would require the combination of airflow, heat loss, atmospheric conditions, and energy performance simulations.
Building Performance Simulation Use Cases
Deploying modelling and simulation tools for building performance can be implemented in various and numerous use cases from the design stage to operation and management of a building. Some of the most common BPS simulation use cases are:
- Facilitating decision-making for designing and installing specific building components. Specifically, simulating the optimal performance for the user’s comfort and energy consumption.
- Creating a baseline for certain energy certification schemes. Comparing the building’s energy consumption baseline to energy-saving to obtain the correct designs for green energy certifications like BREEAM (Liu et al., 2021).
- Creating a bidding virtual prototype to assess different materials, applications, and systems to identify all the possibilities for future refinement.
- Visualising promising energy control strategies to achieve the optimal building performance (GhaffarianHoseini et al., 2017).
- Fine-tuning HVAC system energy performance and coefficients (Kamel & Memari, 2019).
- Visualising optimal energy controls and actions to increase awareness (Robic et al., 2020).
Building Simulation: Challenges and Concerns
Attia (2010) identifies five major challenges in building simulation —(a) interface usability and information management, (b) integration of decision design support and design optimisation, (c) accuracy and ability to simulate detailed and complex building components, (d) integration with other tools in the building design and construction/renovation process, and (e) BIM integration and interoperability. These challenges were echoed and expanded more recently by Hong et al. (2018). as a total of ten challenges which cover the full building life cycle and include zero-net-energy (ZNE) and grid-responsive buildings, as well as urban-scale building energy modelling.
While the BPS challenges Attia (2010) and Hong et al. (2018) present at a high level are not insignificant, at a more granular and operational level, the selection of a BPS tool is also not without challenges. In addition to the level of accuracy and detail, usability and information management, data exchange capacity, database support, interoperability with building modelling, and integration of building design process, Solmaz (2019) highlights the speed (time to implement), cost, and ease of use of BPS tools as significant issues. Given the centrality of BIM in the building and deep renovation process, it is important to highlight specific challenges noted by many studies with respect to the integration of simulations and specifically energy simulations in BIM (Østergård et al., 2016; Hong et al., 2018; Kamel & Memari, 2019; Solmaz, 2019). Challenges include interactions between components, file-related interoperability issues at the file and syntax level, visualisation level, and semantic level, different calculation methods, attribute support, missing data, and data loss between systems (Kamel & Memari, 2019).
Building simulation can provide valuable insights across all the stages of the building and deep renovation life cycle. It may be used for many use cases as it is adaptable to various inputs and it can be deployed based on different needs. Furthermore, there is a plethora of tools and technologies that may support the simulation process. Simulation technology is evolving rapidly with advancements in simulation techniques, software, and hardware. However, most of the simulation applications or auxiliary tools (e.g., BIM and DT) have significant data demands, leading to higher demand for storage and computational resources. As a result, new big data handling solutions must be developed to support the simulation process. In the future, building simulation will undoubtedly be a significant element in the whole cycle of the building; however, both integration and interoperability remain significant challenges that should not be underestimated.
To learn more and download the full open access book, ‘Disrupting Buildings: Digitalisation and the Transformation of Deep Renovation,’ click here.
References
Alor-Hernández, G., & Valencia-García, R. (Eds.). (2017). Current trends on knowledge-based systems. Springer International Publishing.
Attia, S., Hamdy, M., O’Brien, W., & Carlucci, S. (2013). Assessing gaps and needs for integrating building performance optimization tools in net zero energy buildings design. Energy and Buildings, 60, 110–124.
Attia, S. (2010). “Building performance simulation tools: selection criteria and user survey.”.
Bermeo-Ayerbe, M. A., Ocampo-Martinez, C., & Diaz-Rozo, J. (2022). Data-driven energy prediction modeling for both energy efficiency and maintenance in smart manufacturing systems. Energy, 238, 121691.
Bramstoft, R., Alonso, A. P., Karlsson, K., Kofoed-Wiuff, A., & Münster, M. (2018). STREAM—An energy scenario modelling tool. Energy Strategy Reviews, 21, 62–70.
Dimara, A., Anagnostopoulos, C. N., Krinidis, S., & Tzovaras, D. (2021, January). Benchmarking of regression algorithms for simulating the building’s energy. In 2021 IEEE 11th Annual Computing and Communication Workshop and Conference (CCWC) (pp. 94–100). IEEE.
Fanti, M. P., Mangini, A. M., & Roccotelli, M. (2018). A simulation and control model for building energy management. Control Engineering Practice, 72, 192–205.
Fernandez-Antolin, M. M., del Río, J. M., & González-Lezcano, R. A. (2022). Building performance simulations and architects against climate change and energy resource scarcity. Earth, 3(1), 31–44.
GhaffarianHoseini, A., Zhang, T., Nwadigo, O., GhaffarianHoseini, A., Naismith, N., Tookey, J., & Raahemifar, K. (2017). Application of nD BIM Integrated Knowledge-based Building Management System (BIM-IKBMS) for inspecting post-construction energy efficiency. Renewable and Sustainable Energy Reviews, 72, 935–949.
Hong, T., Langevin, J., & Sun, K. (2018, October). Building simulation: Ten challenges. In Building simulation (vol. 11, no. 5, pp. 871–898). Springer.
Kamel, E., & Memari, A. M. (2019). Review of BIM’s application in energy simulation: Tools, issues, and solutions. Automation in Construction, 97, 164–180.
Kampelis, N., Papayiannis, G. I., Kolokotsa, D., Galanis, G. N., Isidori, D., Cristalli, C., & Yannacopoulos, A. N. (2020). An integrated energy simulation model for buildings. Energies, 13(5), 1170.
Laghmich, N., Romani, Z., Lapisa, R., & Draoui, A. (2022, January). Numerical analysis of horizontal temperature distribution in large buildings by thermo-aeraulic zonal approach. In Building simulation (vol. 15, no. 1, pp. 99–115). Tsinghua University Press.
Liu, M., Fang, S., Dong, H., & Xu, C. (2021). Review of digital twin about concepts, technologies, and industrial applications. Journal of Manufacturing Systems, 58, 346–361.
Loonen, R. C., Favoino, F., Hensen, J. L., & Overend, M. (2017). Review of current status, requirements and opportunities for building performance simulation of adaptive facades. Journal of Building Performance Simulation, 10(2), 205–223.
Mahdavi, A. (2020). In the matter of simulation and buildings: Some critical reflections. Journal of Building Performance Simulation, 13(1), 26–33.
Martin, Aleysha K., et al. (2022). “Co-production of a transdisciplinary assessment by researchers and healthcare professionals: a case study.” Public Health Res Pract 10.
Østergård, T., Jensen, R. L., & Maagaard, S. E. (2016). Building simulations supporting decision making in early design—A review. Renewable and Sustainable Energy Reviews, 61, 187–201.
Oucquier, A., Robert, S., Suard, F., Stephan, L., & Jay, A. (2013). State of the art in building modeling and energy performances prediction: A review. Renewable and Sustainable Energy Reviews, 23, 272–288.
Puttur, U., Ahmadi, M., Ahmadi, B., & Bigham, S. (2022). A novel lung-inspired 3D-printed desiccant-coated heat exchanger for high-performance humidity management in buildings. Energy Conversion and Management, 252, 115074.
Robic, F., et al. (2020). Implementation and fine-tuning of the Big Bang-Big Crunch optimisation method for use in passive building design. Building and Environment, 173, 106731.
Shen, R., Jiao, Z., Parker, T., Sun, Y., & Wang, Q. (2020). Recent application of Computational Fluid Dynamics (CFD) in process safety and loss prevention: A review. Journal of Loss Prevention in the Process Industries, 67, 104252.
Solmaz, A. S. (2019). A critical review on building performance simulation tools. Alam Cipta, 12(2), 7–21.
Tan, Y., Peng, J., Luo, Y., Gao, J., Luo, Z., Wang, M., & Curcija, D. C. (2022). Parametric study of venetian blinds for energy performance evaluation and classification in residential buildings. Energy, 239, 122266.
Tian, Z. (2018b). Towards adoption of building energy simulation and optimization for passive building design: A survey and a review. Energy and Buildings, 158, 1306–1316.
Vera, S. (2018). A critical review of heat and mass transfer in vegetative roof models used in building energy and urban environment simulation tools. Applied Energy, 232, 752–764.
Yu, Y. A. (2019). A review of the development of airflow models used in building load calculation and energy simulation. Building Simulation, 12(3).